1. Introduction
Kidneys play a pivotal role in the human body as essential regulators of waste filtration, fluid balance, and electrolyte equilibrium. Kidney failure, characterized by the loss of renal function, stands as a multifaceted and pressing global health concern, impacting millions worldwide.[1] Kidney failure adversely impacts health-related life quality, encompassing physical, emotional, and social well-being.[2] Furthermore, prevailing trends indicate an increasing incidence of chronic and end-stage kidney disease.[3] Thus, there have been a demand for studies focusing on this issue from various perspectives, particularly those involving the prediction of kidney failure occurrence.
The prediction of kidney failure necessitates the incorporation of numerous variables. Consequently, developing a comprehensive predictive program is challenging due to its inherent complexity. Machine learning (ML) has been increasingly recognized as a viable approach to enhance the precision of kidney failure prediction and has already demonstrated its potential in predicting various diseases.[4-6] In this context, our specific objective was to predict kidney failure utilizing nationwide, readily accessible regular health check-up data from individuals in South Korea by the development and assessment of multiple ML models to identify the optimal predictive model. Additionally, while age is commonly assumed as a prominent factor in predicting kidney failure, this study endeavored to provide a comprehensive perspective on the relevance of other factors and to compare their significance with that of age.[7, 8]
2. Methods
We used national, large-scale, and general-population-based cohort data from South Korea; The National Health Insurance Service-National Sample Cohort (NHIS-NSC; total n=973,303).[9-11] The cohort was built and provided by the NHIS of South Korea based on participants aged 20 years and above between 1 January 2002 and 31 December 2013. The results of nationwide regular health check-ups were used. This cohort study has the following advantageous characteristics: (1) The Korean government has been conducting mandatory regular health check-ups on all Koreans aged 20 years and above every year; (2) It records whether the individual has been diagnosed with kidney failure every year; (3) To ensure confidentiality, the Korean government anonymized all individual-related information.[9] Participants with insufficient information, who died, or with unknown kidney failure status were excluded. Figure S1 illustrates the data selection process from NHIS-NSC. The research protocol was authorized by the Institutional Review Board of Kyung Hee University and NHIS of Korea, and written informed consent was waived by the ethics committee, owing to the routinely collected data.
To develop a ML model capable of predicting kidney failure within five years, we acquired several variables as follows: age (continuous); sex (male and female); household income (basic livelihood recipient, 0-9, 10-19, 20-29, 30-39, 40-49, 50-59, 60-69, 70-79, 80-89, and 90-100 percentile); region of residence (rural and urban); body mass index (BMI; continuous)[12]; systolic blood pressure (continuous); diastolic blood pressure (continuous); fasting blood glucose (FBG; continuous); serum total cholesterol (continuous); hemoglobin (continuous); aspartate transaminase (continuous); alanine transaminase (continuous); γ-glutamyl transpeptidase (continuous); history of kidney related diseases (Table S1); history of diabetes mellitus, stroke, and hypertension; smoking status (never, ex-, and current smoker); alcoholic drink consumption (<1, 1-2, 3-4, and ≥5 days per week); and moderate-to-vigorous physical activity (0, 1-2, 3-4, 5-6, and 7 days per week). All included features for the artificial intelligence (AI) model are summarized in Table S2.
None of the patients were directly involved in designing the research questions or conducting the research. Patients were not asked for advice on the interpretation or writing of the results. There were no plans to involve patients or the relevant patient community in the dissemination of study findings.
In this study, we split the multiplied NHIS-NSC dataset (n=1,048,422) into training (n=838,562) and testing (n=209,860) datasets with a ratio of 8:2 in a stratified fashion. Table S3 summarizes the distribution of training and testing data. The testing set was used exclusively for conducting an independent test of our developed AI models and was not utilized for training or internal validation.
We first performed five-fold cross-validation using the training data to confirm its generalizability. The training dataset (n=838,562) was randomly shuffled and stratified into five equal groups, four groups were selected for training the model, and the remaining group was used for internal validation. This process was repeated five times by shifting the internal validation group for the cross-validation. Since the number of kidney failure data (n=4,844; 1.15%) was even lower than that of non-kidney failure data (n=416,077; 98.75%), we up-sampled the kidney failure data using a synthetic minority oversampling technique during the model update.[13] By balancing the two groups, we aimed to prevent bias toward the kidney failure group.
We initially applied six distinct ML algorithms: extreme gradient boosting, gradient boosting machine (GBM), light gradient-boosting machine (LightGBM), random forest, adaptive boosting, and logistic regression (LR). Subsequently, we identified the top-performing three models (GBM, LightGBM, and LR) among the six models and applied an ensemble approach by considering every possible combination. For the robustness of our model, an ensemble model was adopted.
We selected a best-achievement model among the ensemble models, which was the combination of LightGBM and LR. To attenuate age-centric bias in the predictive model,[7] we undertook an ensemble approach combining two ensemble models: the model with all features and the model without age. Fig. 1 illustrates the double-ensemble model designed to predict kidney failure within five years after receiving regular health check-ups. Each single-ensemble model provided the probabilities: pall from the general model and pex_age from the model excluding age. Subsequently, pall and pex_age were multiplied by model weight values, wall and wex_age, respectively. To find the weight values, wall and wex_age, we investigated the prediction performance by changing wall and wex_age. Finally, the sum of the two multiplications was considered as the final probability for kidney failure.
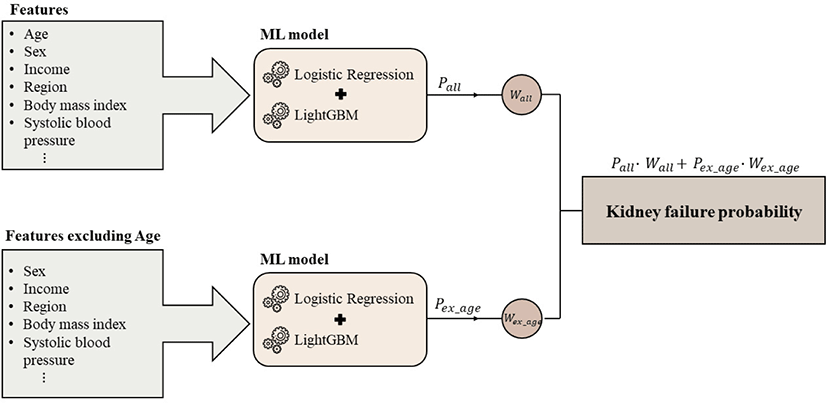
The evaluation of model performance was based on five metrics, including sensitivity, specificity, accuracy, balanced accuracy, and area under receiver of characteristics (AUROC) from five-fold cross-validation. Especially due to severe data imbalance, we focused on balanced accuracy and AUROC for evaluation metrics of the main model (single-ensemble model with all features). Based on the final model, we further presented the relative importance of individual features, listing features in the order they contributed to the prediction of kidney failure occurrence within five years after receiving regular health check-ups.
We implemented the models using Python (version: 3.9.16; Python Software Foundation, Wilmington, DE, USA) with TensorFlow (version: 2.9.1), Keras (version: 2.9.0), NumPy (version: 1.21.5), Pandas (version: 1.4.4), Matplotlib (version: 3.5.2), and Scikit-learn (version: 1.0.2). All statistical analyses were performed using SAS (version 9.4; SAS Institute Inc., Cary, NC, USA).[14, 15]
3. Results
There are 4,844 participants with kidney failure (mean age 60.1 [standard deviation, 14.0] years; 2,274 [46.9%] male) and 416,077 those without kidney failure (mean age 50.2 [standard deviation, 14.6] years; 217,345 [52.2%] male). Table 1 depicts a comparison of variables included in the NHIS-NSC.
Table 2 summarizes the five-fold cross-validation result in comparison to other ML models. Regarding the balanced accuracy and AUROC, when considering only single models, the most noteworthy performance was demonstrated by LR (balanced accuracy, 0.691 and AUROC, 0.754), LightGBM (balanced accuracy, 0.680 and AUROC, 0.740), and GBM (balance accuracy, 0.680 and AUROC, 0.739). To further elevate prediction performance, we investigated the ensemble approach using the possible combinations of the top three single models: LR, LightGBM, and GBM. The results showed that the ensemble model with the combination of LightGBM and LR provided the highest performance than any other single or combination models. For LightGBM, we found the following optimized hyper-parameters: number of tree estimators with 300, learning rate with 0.01, the tree depth explicitly with 2, and a minimal amount of data in one leaf with 2. For LR, penalty norm with L2 (ridge), the inverse of regularization strength with 10, and maximum number of iterations with 100.
Table 3 summarizes the prediction accuracy according to the values of wall and wex_age. The results showed that when the weights of the model with all features and the model without the age feature were assigned in a ratio of 7:3 (wall = 0.7 and wex_age = 0.3), finally, it achieved the highest balanced accuracy of 0.691 and AUROC of 0.754. Table S4 presents the results of the five-fold cross-validation comparison for the model considering all features, the model excluding age, and the final model combining the two.
Table 4 summarizes the model performance in the test dataset from the isolated testing dataset (n=209,860) from multiplied NHIS-NSC. The testing data results also show that the ensemble of models, which includes all features and excludes age, consisting of LightGBM and LR, provided the highest values for balanced accuracy of 0.692 and AUROC of 0.754. Similar to the cross-validation results, the ensemble model provided the best performance in the testing datasets. The similarity between the results from the cross-validation and the testing data indicates that overfitting or underfitting was minimal in the model.
To analyze the effect of each feature to predict kidney failure occurrence, we performed the feature importance analysis to confirm the contribution of each feature. Fig. 2 shows the ranked normalized feature importance from the final ensemble model with the combination of LightGBM and LR. The results show that age was the top contributor to predict kidney failure occurrence within five years. The next important features were BMI, FBG, diastolic blood pressure, and systolic blood pressure. Table S5 summarizes the complete ranked normalized feature importance values.
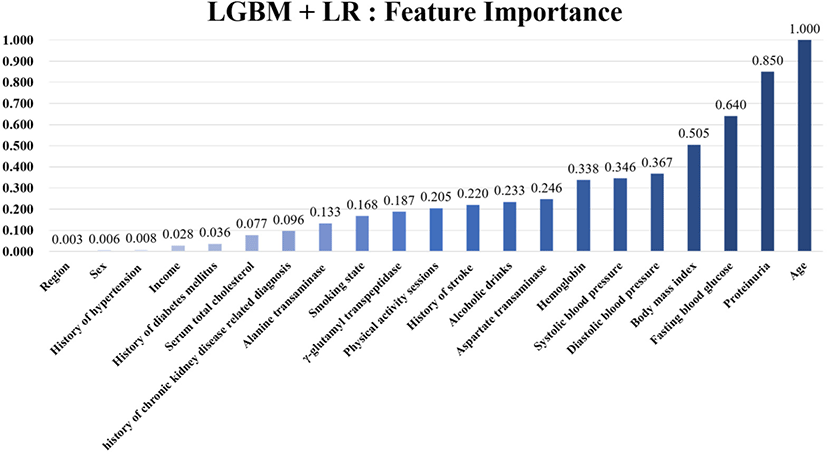
Our proposed ensemble model was deployed on our own public website (http://ai-wm.khu.ac.kr/KidneyFailure/), so that kidney failure onset within five years can be predicted based on regular health check-up data. The deployed web application, which provides results for prediction of kidney failure onset, is shown in Fig. 3. The web interface for entering information on 20 features from regular health check-up data is shown in Fig. 3. After entering the information in the web application, a user can immediately obtain the results for prediction of kidney failure onset with its probability, as shown in Fig. 3. In the web application, the features input by a user are encoded to the website server and immediately deleted upon generation of the prediction result, so that there is no risk of exposing information. In addition, there is no need to enter any information that would be regarded as private.
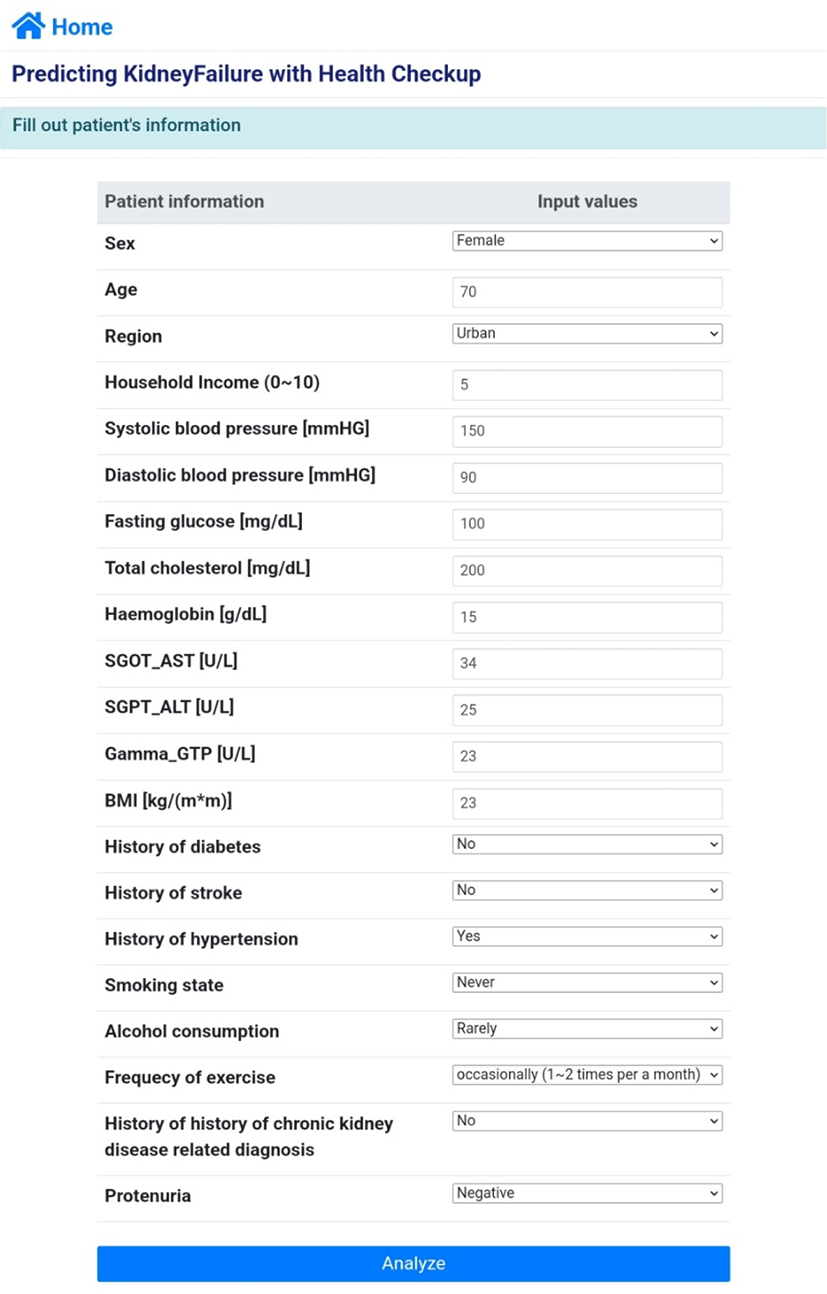
4. Discussion
To our knowledge, this is the first ML model capable of predicting kidney failure with only regular health check-up data. In this investigation, we employed ML models to predict the onset of kidney failure within a five-year timeframe. We evaluated each performance of 10 distinct models, including six single models and four ensemble models. Among the models, the ensemble model consisting of Light GBM and LR, which includes all features and excludes age, found the highest performance, with a balanced accuracy of 0.691 and an AUROC of 0.754 on the validation dataset and a balanced accuracy of 0.692 and an AUROC of 0.754 on the testing dataset. Feature importance analysis revealed that the five most critical predictors of kidney failure were age, FBG, systolic blood pressure, hemoglobin, and diastolic blood pressure. This feature importance analysis conducted through our model approaches highlighted features that clinicians should pay attention to and consider as risk factors when assessing the likelihood of kidney failure occurrence.
Previous research has explored the utilization of ML models for predicting kidney-related disease, aligning with our study’s focus. Along with the positive outlook for the introduction of ML in the prediction of kidney failure,[16] several studies have demonstrated the potential of ML in predicting kidney failure. For instance, one study suggested that the ML model could predict pediatric acute kidney injury (AKI) up to 48 hours earlier than the previous diagnosis guidelines with an AUROC of 0.89 for predicting severe AKI.[17] Another study used unsupervised ML algorithms to subtype patients with CKD to enable more effective prediction by taking into account different key risk factors for each subgroup.[18] These studies as well as ours support the potential of the ML models for the prediction of kidney failures and the continued need to improve the models.
As discussed above, this study shows the continuous importance of understanding what factors are associated with the risk of kidney failure. Conventionally, age has been consistently acknowledged as a key determinant of various health issues, including kidney failure.[19] This study, while proving it, also identifies BMI and FBG as crucial factors for kidney health. A study suggested that excess weight is a common, strong, and modifiable risk factor for CKD and end-stage renal disease, showing similar results with our study.[20] Another study showed higher BMI was significantly associated with an increased risk of CKD development in hypertensive patients with normal kidney function.[21] Furthermore, a study, concluding with similar features as important, showed the association of age and BMI with kidney function and showed that a BMI of 30kg/m2 or more is associated with rapid loss of kidney function in patients with estimated glomerular filtration rate (eGFR) of at least 60mL/min per 173 m2.[22] In summary, both our study and prior research show that there is clear evidence that obesity (BMI over 30) is associated with an increased risk of kidney failure. There were studies suggesting other significant features for the diagnosis and prognosis of CKD, such as packed cell volume, specific gravity, red blood cell counts, and albumin, while there were similar features such as hemoglobin.[23] These studies show that there is a need to explain the mechanisms of suggested important features, especially for the commonly reported features in several studies.
Age, BMI, and FBG emerged as pivotal factors in predicting kidney failure, likely due to their association with diabetes and hypertension, both of which profoundly influence cardiovascular health and kidney function.[24] In contrast, factors such as sex, physical activity levels, and a history of stroke may exert a less direct influence on kidney failure or potentially affect kidney health indirectly through cardiovascular mechanisms.[25]
The primary mechanism linking BMI and FBG levels to kidney failure is their connection to type 2 diabetes.[26] High BMI often correlates with higher blood pressure and an increased likelihood of diabetes which can contribute to kidney damage. Notably, hypertension and diabetes are the leading causes of CKD according to the US Department of Health and Human Services.
FBG level is also closely associated with hypertension which can lead to the constriction of small arteries and arterioles supplying blood to the kidneys. This can compromise the eGFR. Prolonged hypertension also exerts mechanical stress on blood vessel walls, causing vascular damage. This impairment in vascular integrity triggers inflammation and oxidative stress, exacerbating kidney deterioration. Additionally, elevated blood glucose levels can damage blood vessels within glomeruli, resulting in albuminuria, an early indicator of kidney damage.[27, 28]
Our study provides valuable insights for both clinicians and policymakers. Primarily, it underscores the significance of blood glucose and blood pressure levels in predicting kidney failure, enabling doctors to emphasize and monitor their association with kidney failure. Moreover, our model allows for the quick and efficient prediction of kidney failure using simple, regular check-up results, which can significantly aid in risk reduction through early intervention. Furthermore, given the high performance of the model developed in this study, it holds potential for commercialization as an effective predictive tool with further research and refinement. For policymakers, it is important for them to raise awareness of the contribution of features to the general population. By understanding the significance of these key features in contributing to kidney failure, individuals can proactively monitor these factors, take preventive measures, and improve their overall health through early response.
This study has several limitations. First, although our dataset encompasses over a million cases, it primarily represents a limited Asian population. There may be unique features specific to Koreans that influence the predictive outcomes. Consequently, there is a need to assess the performance of our model using a large-scale, international external validation dataset. Second, our study did not establish a causal link between the features utilized for model training and the onset of kidney failure. Further research is required to elucidate the biological mechanisms underlying the relationships between the selected features and their influence on kidney failure.
Despite these limitations, our study provides several significant contributions. First, we employed a substantial cohort of Korean population data in our study. We utilized results from mandatory annual health check-ups imposed by the Korean government on all Koreans aged 20 and above, which ensured a robust dataset size. Utilizing a large sample size allowed us to mitigate the risk of overfitting and maintain generalizability.[29] Second, we utilized data from routine health check-ups that are conducted regularly, independent of clinical or environmental settings. This ensures the feasibility of conducting tests with high accessibility. Third, by incorporating a diverse array of features from the dataset, our study was able to offer insights into the relative importance of numerous features. This enables clinicians to identify and compare the significance of features relevant to their needs. Lastly, this model holds the potential to aid in the early detection of kidney failure. Both individuals and clinicians can assess the risk of kidney failure using only annual health check-up data, enabling prompt medical intervention. Considering the importance of early diagnosis in kidney failure, it is clear that our study offers significant value.[30]
5. Conclusion
ML is an innovative field that offers the potential to predict kidney failure with ease. In this study, we utilized a variety of features to develop several ML-based models for predicting kidney failure within five years. The ensemble models, which includes all features and excludes age, consisting of LightGBM and LR demonstrated the highest performance (validation dataset: AUROC, 0.7541; test dataset: AUROC, 0.7538). Additionally, age, BMI FBG, diastolic blood pressure, and systolic blood pressure emerged as significant features based on their relative feature importance rankings in this model. This study holds significance as it demonstrates the feasibility of creating a highly accurate predictive model using simple and routine tests. While current national clinical guidelines for kidney failure may be cautious about adopting predictive models, such models could serve as valuable tools for preventing kidney failure in the Korean population in the future.
The study emphasizes the potential of machine learning models in predicting kidney failure occurrences within five years after annual health check-up data, encouraging broader implementation of such models to enhance public health and decrease the prevalence of kidney failure by preventive intervention.