1. Introduction
The global crisis triggered by events such as the COVID-19 pandemic has placed significant strains on healthcare systems.[1] The overwhelming demand for COVID-19 care has further exacerbated these challenges, leading to capacity constraints in hospitals' ability to accommodate non-COVID-19 patients.[2] This has resulted in shortages of healthcare personnel and increased concerns among patients regarding the delivery of healthcare services.[3] Moreover, the implementation of social distancing measures and lockdown policies has not only created isolation among patients but has also led to decreased healthcare accessibility.[4] This has led to a significant reduction in access to public health and healthcare services, especially for patients who need to manage chronic conditions.[5] As healthcare facilities reach their capacity, the risk of chronic disease increases, and the socioeconomic impact becomes more severe, the need for alternative healthcare models is growing.[6-8] To this end, it is essential to comprehensively understand the impact of the COVID-19 pandemic on various population groups and establish policies tailored to each group.[9]
Empirical evidence from prior research highlights that the COVID-19 pandemic disproportionately affected specific groups with poor socioeconomic status, such as the elderly and ethnic minorities, as well as those with specific diseases.[10, 11] In particular, type 2 diabetes is the most prevalent comorbidity associated with severe acute respiratory syndrome coronavirus 2 (SARS-CoV-2) infection.[12] Individuals with diabetes have exhibited heightened mortality rates upon contracting COVID-19 in comparison to those without the condition.[13] Additionally, diabetes, being a chronic ailment, exhibits a consistent upward trajectory on a global scale and is known to give rise to a multitude of complications.[14] Therefore, the enduring patterns in diabetes must be comprehensively understood and the ramifications of the COVID-19 pandemic must be evaluated, including the identification of populations susceptible to this disease.
Previous studies have established a link between diabetes and COVID-19, suggesting that people with diabetes may have a higher risk of severe outcomes like complications and an increased risk of death if they are infected with COVID-19; however, these studies were constrained by limited sample sizes and restricted temporal scopes.[15, 16] To address these limitations, our study delineated diabetes trends over a 24-year period, distinguishing between the periods before (1998–2019) and during the pandemic (2020–2021). Ultimately, we aimed to study trends in the prevalence of diabetes in South Korea over a long period of 24 years including the COVID-19 pandemic, to identify potential risk factors and vulnerable populations associated with diabetes.
2. Methods
Our study conducted a comprehensive analysis that incorporated various socioeconomic variables. To accomplish this, we leveraged extensive national data from the Korea National Health and Nutrition Examination Survey (KNHANES) conducted by the Korea Disease Control and Prevention Agency (KDCA) between 1998 and 2021.[17, 18] The sampling frame of KNHANES utilized the most recent population and housing census data available at the time of sampling design as the basic sampling frame to ensure that a representative sample is randomly selected from the target population of people aged 1 year and older living in the Republic of Korea. Our study participants were selected if they were aged ≥30 years based on criteria from previous diabetes studies, and their information included age, sex, region of residence, body mass index (BMI) group, education, smoking status, and household income.[19] To compare the prevalence of type 2 diabetes both before and during the COVID-19 pandemic, a nationally representative sample of 135,671 participants was selected. The survey spanned 24 years, with participant counts per year grouped as follows: 62,163 in 1998–2005; 14,598 in 2007–2009; 15,610 in 2010–2012; 13,440 in 2013–2015; 20,601 in 2016–2019; 4,613 in 2020; and 4,646 in 2021 (Fig. S1). This grouping by year follows the sample design guidelines of KNHANES, which was conducted by KCDA, with 2020 and 2021 set aside to further analyze the impact of the COVID–19 pandemic.
The research protocol was approved by the Institutional Review Boards of the KDCA (2007-02CON-04-P, 2008-04EXP-01-C, 2009-01CON-03-2C, 2010-02CON-21-C, 2011-02CON-06-C, 2012-01EXP-01-2C, 2013-07CON-03-4C, 2013-12EXP-035C) and by the local law of the Act (Article 2, Paragraph 1) and Enforcement Regulation (Article 2, Paragraph 2, item 1) of Bioethics and Safety Act, from Korean government. Written informed consent was obtained from all participants prior to participation. In addition, KNHANES provides access to public data, which can serve as a useful resource for a variety of epidemiologic studies. Furthermore, our study involved human participants and was conducted in compliance with relevant guidelines/regulations and the Declaration of Helsinki.
The primary goal of our study was to investigate the annual change in the prevalence of diabetes over a period of 24 years, spanning from 1998 to 2021. Participants were asked a targeted question associated with their history of diagnosed diabetes, specifically inquiring, “Have you ever been diagnosed with diabetes by a doctor?”.[20] Based on their responses, we collected data on various potential determinants associated with the development of diabetes.
The covariates used in this study include age (30–39, 40–49, 50–59 and ≥60 years), sex, region of residence (urban and rural),[21] education level (elementary school or lower, middle school, high school, and college or higher education), household income (lowest, second, third, and highest quartile), smoking status (current, ex-, and non-smoker), and BMI group (underweight, normal weight, overweight, and obese). BMI values were categorized into underweight (<18.5 kg/m2), normal weight (18.5–22.9 kg/m2), overweight (23–25 kg/m2), and obese (≥25.0 kg/m2) categories according to Asian-Pacific guidelines.[22, 23] These covariates were selected for determinants related to type 2 diabetes development.
The results of our study were conveyed using quantitative data expressed as weighted proportions with 95% confidence intervals (CIs) or crude numbers with percentages. To compare and analyze the estimates of each related factor both before and during the COVID-19 pandemic, weighted multivariate regression model analyses were conducted with weighted odds ratios (ORs) and 95% CIs.[24, 25] In our cross-sectional study, we evaluated the prevalence of type 2 diabetes, a binary dependent variable, using logistic regression. This method reliably converges by maximizing the log-likelihood function, allowing us to simultaneously assess the impact of multiple independent variables and adjust for confounding factors in a multivariate analysis. The logistic regression model's advantages include its ability to adjust for confounding variables, ensure convergence, provide intuitive interpretation, and avoid the issue of overdispersion when using ORs. This approach enabled us to identify significant predictors and evaluate trends in diabetes prevalence at a specific time point.[26, 27] The prevalence of diabetes was calculated by analyzing data from the KNHANES from 1998 to 2021 and categorizing the data by year. To ensure a precise estimation, a weighted complex sampling analysis was utilized. Linear regression and binary logistic regression models were used to calculate the ORs with 95% CIs or β-coefficients with 95% CIs. To enhance the reliability of the findings, stratification analysis was conducted, accounting for variables such as age, sex, region of residence, education, household income, smoking status, and BMI in all regression models. Moreover, the ratio of ORs (RORs) was calculated to estimate the interaction term of each risk factor and identify groups that were more vulnerable to diabetes for each variable.[28] Overall, this approach aimed to provide a comprehensive and robust assessment of the impact of the COVID-19 pandemic on the prevalence of diabetes and identify the factors that contribute to vulnerability to these conditions.
The KNHANES data is designed with a rolling sampling method to ensure similarity across samples in each wave. This approach compensates for the limited sample size of annual data by combining data from multiple waves. Default weights, considering factors such as the survey year, region, and variables, are initially set. These weights are then adjusted to reflect both the sampling rate and the response rate. Subsequently, the weights are calibrated against the total number of households and the population. This calibration enhances the accuracy and representativeness of the estimates concerning the population's health behaviors and the prevalence of chronic diseases. For this study, sample weights were derived by multiplying the default weights by the proportion of the survey population in each year, effectively compensating for any differences in the timing of sampling and survey. For statistical analyses, our study used SAS software (version 9.4; SAS Institute, Cary, NC, USA), with a two-sided test, and a P-value ≤0.05 was considered statistically significant.[25]
3. Results
From 1998 to 2021, a total of 231,264 participants took part in the KNHANES surveys. However, based on the cohort age criteria of many previous studies on type 2 diabetes, this study excluded individuals aged 0-29 years from the cohort. [29, 30] We further excluded missing values for household income and weights, resulting in 135,671 individuals included in the final study, with the following distribution of characteristics: age (30–39 years, 24.20% [95% CI, 23.98–24.43]; 40–49 years, 23.87% [95% CI, 23.64–24.10]; 50–59 years, 19.89% [95% CI, 19.68–20.11]; and ≥60 years, 32.03% [95% CI, 31.78–32.28]) and sex (male, 44.84% [95% CI, 44.57–45.10] and female, 55.16% [95% CI, 54.90–55.43]). The baseline characteristics of the study population in terms of both crude and weighted rates are presented in Table 1.
Table 2 and Fig. 1 display the diabetes prevalence data along with supplementary data comparing the periods before and during the pandemic from 1998 to 2021. The number of patients diagnosed with diabetes consistently increased overall from 5.61% (95% CI, 5.36–5.86) in 1998–2005 to 10.61% (95% CI, 9.50–11.72) in 2020 and 11.61% (95% CI, 10.36–12.86) in 2021. However, the trend in diabetes prevalence did not increase significantly before and during the pandemic (0.084 [95% CI, 0.069–0.098] and 0.114 [95% CI, 0.046–0.181], respectively).
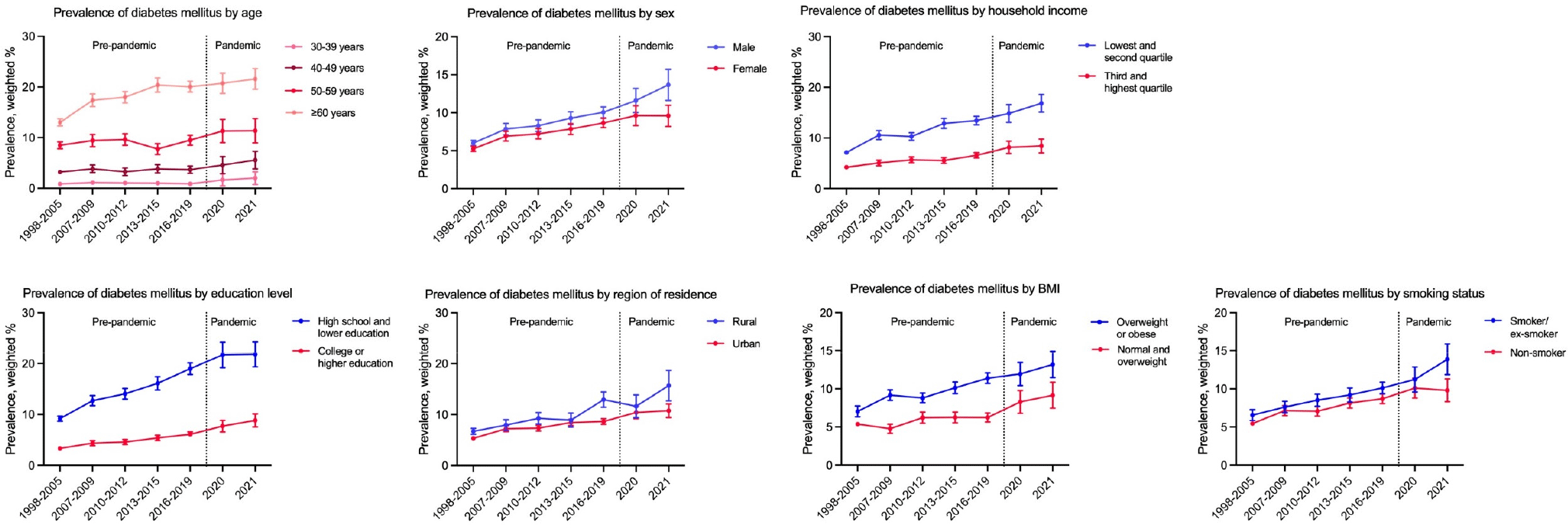
Table 3 shows the pandemic-related factors in vulnerable groups of patients with diabetes. Using the 30–39-year age group as a reference, the overall ORs (95% CI) in each age group were as follows: 40–49 years, 3.67 (95% CI, 3.12–4.32); 50–59 years, 9.76 (95% CI, 8.41–11.34); and ≥60 years, 21.05 (95% CI, 18.21–24.34). In terms of region of residence, rural areas were associated with an OR of 1.43 (95% CI, 1.33–1.53) compared to urban areas. In terms of education level, individuals with a high school or lower education demonstrated an OR of 3.03 (95% CI, 2.88–3.18) compared to those with college or higher education. Regarding household income, the lowest and second quartiles were associated with an OR of 2.10 (95% CI: 2.00–2.21) compared to the third and highest quartiles. When examining lifestyle factors such as smoking status and BMI group, current and ex-smokers had an OR of 1.97 (95% CI,1.88–2.08) compared to non-smokers, while individuals classified as overweight or obese based on BMI had an OR of 2.75 (95% CI, 2.62–2.88) compared to those classified as underweight or having a normal BMI. Furthermore, upon examining changes in each factor before and during the pandemic, we found that the difference in diabetes prevalence between the overweight/obese and normal/underweight groups was significantly reduced during the pandemic compared to before the pandemic, with a difference of 0.82 (95% CI, 0.69–0.96).
As a result, elderly individuals, male sex, those residing in rural areas, those with lower education levels and income levels, current and ex-smokers, and individuals classified as being overweight or obese based on BMI were more vulnerable to diabetes. However, the vulnerability profiles of these groups did not change significantly before or during the pandemic. The results of a more detailed analysis by year are presented in Table S1.
4. Discussion
We analyzed the trends in diabetes prevalence before and during the COVID-19 pandemic using data from the KNHANES database, a nationally representative survey of over 130,000 South Koreans conducted over a 24-year period from 1998 to 2021. During the entire study period, including the COVID-19 pandemic, a steady increase in diabetes prevalence was observed each year. Older age, male sex, rural residence, lower education, low economic status, smoking, and overweight or obesity were risk factors for diabetes. When comparing prevalence trends before and during the pandemic, we found a slight increase in the overall prevalence slope during the pandemic compared to before the pandemic, although this was not statistically significant. However, in BMI groups, there was a decrease in the difference in diabetes prevalence between the overweight/obese group and the normal/underweight group during the pandemic compared to before the pandemic.
Over the 24-year period from 1998 to 2021, we observed a consistent and striking trend: the prevalence of diabetes has more than doubled. Furthermore, during the COVID-19 pandemic, certain demographic groups were identified as particularly vulnerable to diabetes. These groups included older individuals, male sex, rural residents, individuals with lower educational levels, those with reduced household incomes, smokers, and individuals classified as obese or overweight based on their BMI. This finding suggests that segments of the population with limited healthcare access may face increasing challenges during the pandemic.[31]
The rising prevalence of diabetes with increasing age underscores the likelihood of continued increases in the prevalence of diabetes as we enter an aging era. Additionally, diabetes is influenced by an array of factors, including dietary habits and physical activity levels.[32, 33] Studies have demonstrated that unhealthy dietary habits and sedentary lifestyles significantly impact diabetes prevalence.[34, 35] Diverse dietary patterns, characterized by a focus on high-sodium and high-fat foods, coupled with a lack of physical activity in past Korean society, have contributed to a yearly increase in the proportion of individuals classified as overweight or obese.[36-38] Given the substantial influence of obesity rates on diabetes, it is imperative to enhance individual awareness regarding dietary habits and exercise.[39]
Another finding of our study was that the difference in diabetes prevalence rates between the overweight/obese and normal/underweight groups decreased during the pandemic compared to before the pandemic. The decrease in the diabetes prevalence odds ratio for the overweight/obese population during the pandemic may be due to a decrease in visits to healthcare facilities, which may have resulted in inadequate diabetes screening.[40] This is likely because lifestyle changes due to the pandemic led to reduced physical activity and changes in health behaviors, which may have been a major factor in reducing the disparity in diabetes prevalence between overweight/obese and normal/underweight populations.[41] These findings have important implications for planning public health strategies and preventive measures.
Our study had several limitations. Firstly, diabetes is a disease that is related to various lifestyle factors, including exercise. However, due to the lack of exercise data from 1998 to 2004, we were unable to consider exercise as a factor in our study. To overcome this limitation, we included other lifestyle-related factors such as BMI and smoking in our analysis. In future studies, adding exercise-related factors will help elucidate these relationships and develop better measures for diabetes management and prevention. Second, after the pandemic, many patients were reluctant to visit the hospital due to concerns about infection, which may have led to an underestimation of cases associated with diabetes. Third, the data in our study depended on self-reported data, which could have introduced recall and social biases. Fourth, our study studied trends in diabetes prevalence over the past 24 years, including the COVID-19 pandemic, to identify populations vulnerable to diabetes, but could not accurately find the logical link between COVID-19 and diabetes. Nevertheless, the finding that diabetes prevalence did not change significantly in South Korea during the COVID-19 pandemic may provide important baseline data for assessing the impact of the pandemic on chronic disease prevalence. Our results can guide future research by analyzing the effectiveness of healthcare policies and suggesting specific directions for the development and strengthening of chronic disease management strategies. This information is valuable not only for understanding the implications of Type 2 diabetes but also for informing broader public health response and management strategies. Fifth, these data involved only South Korean populations, which may limit the generalizability of our findings on diabetes prevalence to global populations. Further studies involving multiple ethnicities and countries are required to overcome these limitations. Finally, our study was set as a cohort of people over 30 years old, which is the standard age for diabetes in adults in many existing studies, excluding the population aged 0–29 years. Although our study focuses on type 2 diabetes, this age limitation may limit our understanding of the full spectrum of diabetes, including early-stage diabetes onset and pre-diabetes. Therefore, there may be limitations in missing information on changes in diabetes prevalence and prevention strategies, especially among young people. In addition, missing values in household income and weighted value were additionally excluded, resulting in a final study population of 135,671 people. As a result, we excluded only 0.07% as missing during the data preprocessing process for the final analysis, but there is a possibility that deviations may occur due to this.
Despite these limitations, the strength of our study lies in the use of a large, nationally representative data spanning 24 years to monitor diabetes trends. We meticulously analyzed variations across diverse demographic groups, both before and during the COVID-19 pandemic. This approach will enable the development of tailored policies for diabetes management and prevention that account for factors such as age, sex, education, income, and region of origin. Furthermore, our findings provided insights into how diabetes prevalence evolves during global crises such as the COVID-19 pandemic, informing response and prevention strategies.[42]
According to our study, over time, vulnerable groups in terms of medical access, such as rural residents, those with lower education levels, and those with lower household incomes, are at risk of diabetes due to insufficient diagnosis. Therefore, the government should formulate relevant policies to address these needs. Additionally, lifestyle factors, such as smoking status and obesity, have a negative impact on the prevalence of diabetes. Consequently, strategies to improve these factors are necessary to reduce the prevalence of diabetes. Finally, many people tend to avoid healthcare visits for fear of infection during the pandemic. This, coupled with a reduction in outside activity, makes it difficult to get an accurate disease diagnosis. Therefore, adequate countermeasures are needed to address these concerns during the pandemic, to ensure that individuals have access to appropriate healthcare despite concerns about infection, and to ensure that they can get an accurate disease diagnosis even when outside activity is limited.
5. Conclusion
In this study, we identified an increasing trend in diabetes prevalence every year before and during the pandemic. Additionally, we found that older individuals, male sex, rural residents, those with lower education levels and lower household income levels, smokers, and those who were overweight/obese had a higher prevalence of diabetes. Therefore, individual awareness and behavioral changes are crucial. Moreover, national-level policies are necessary to address the issue of insufficient diagnosis among vulnerable groups with limited access to healthcare benefits, including individuals residing in rural areas, those with lower education levels, and those with lower household incomes. Although several statistical analyses have shown that the COVID-19 pandemic has not had a significant impact on diabetes prevalence, the reduction in the difference in diabetes prevalence between overweight/obese and normal/underweight groups during the pandemic is an important finding. This means that special attention should be paid to overweight/obesity groups when developing diabetes management and prevention strategies, to gain a deeper understanding of the impact of changed health behavior during the pandemic on chronic disease management, and to implement appropriate public health interventions. It provides important information to develop.